Unravelling Risk & Alpha
Case Study
Executive Summary
Fundamental managers are not focused on quantitative factors, but their strategies inevitably take positions aligned with various risk factors. We show how a constrained portfolio rebalancing can reduce extraneous factor risk and increase stock-specific risk, thus increasing alpha among managers who excel at fundamental stock picking. We demonstrate the application of these techniques on a real portfolio.
Introduction
Fundamental managers, or bottom-up stock pickers, are managers who look at underlying business fundamentals and engage in robust security analysis to determine which stocks to include in their portfolio. They look at a company’s balance sheet, their earnings reports, business fundamentals, competitive moats, industry reports, economic trends, management track record and more to get a sense of the company’s future health. They compare their own analysis of a company with the valuation given to the company by the stock market, and make a decision as to whether or not it is worth including in their portfolio. The better the risk-reward, the larger they will size the position.
As they make decisions about the number and quantity of stocks that they will include in their portfolio, managers are guided by a variety of risk protocols. For example, there’s typically a limit on the percentage of the portfolio that a portfolio manager can put into one stock. Some managers may have minimum or maximum sector weightings, or rules about geographic diversification. The purpose of these rules is to avoid exposure to unexpected risk. For example, airline stocks might be cheap in a given year, but if a portfolio manager puts all of his or her assets into airline stocks and war in the Middle East breaks out, then the portfolio will be destroyed. To avoid overexposure to such risks, managers are encouraged to diversify in markets that are not all directly connected to each other.
Quantitative factor models provide another perspective on mitigating unwanted systematic risk. Tools built around factor analysis can be used to ensure that unwanted risk factors are lessened in order to put the emphasis of the portfolio on the stock-specific alpha being generated by the manager.
We are going to look at a portfolio that was exposed to unnecessary risk factors and demonstrate how factor analysis can rebalance the portfolio to emphasize the stock specific risk, thereby giving fundamental bottom- up stock pickers a comparative advantage. See the Technical Appendix for more information on the factor model and optimizer that we are using in this case
The Case Study
In this case study, we are looking at a multi-hundred billion dollar global fundamental investment portfolio from 2007-2015.
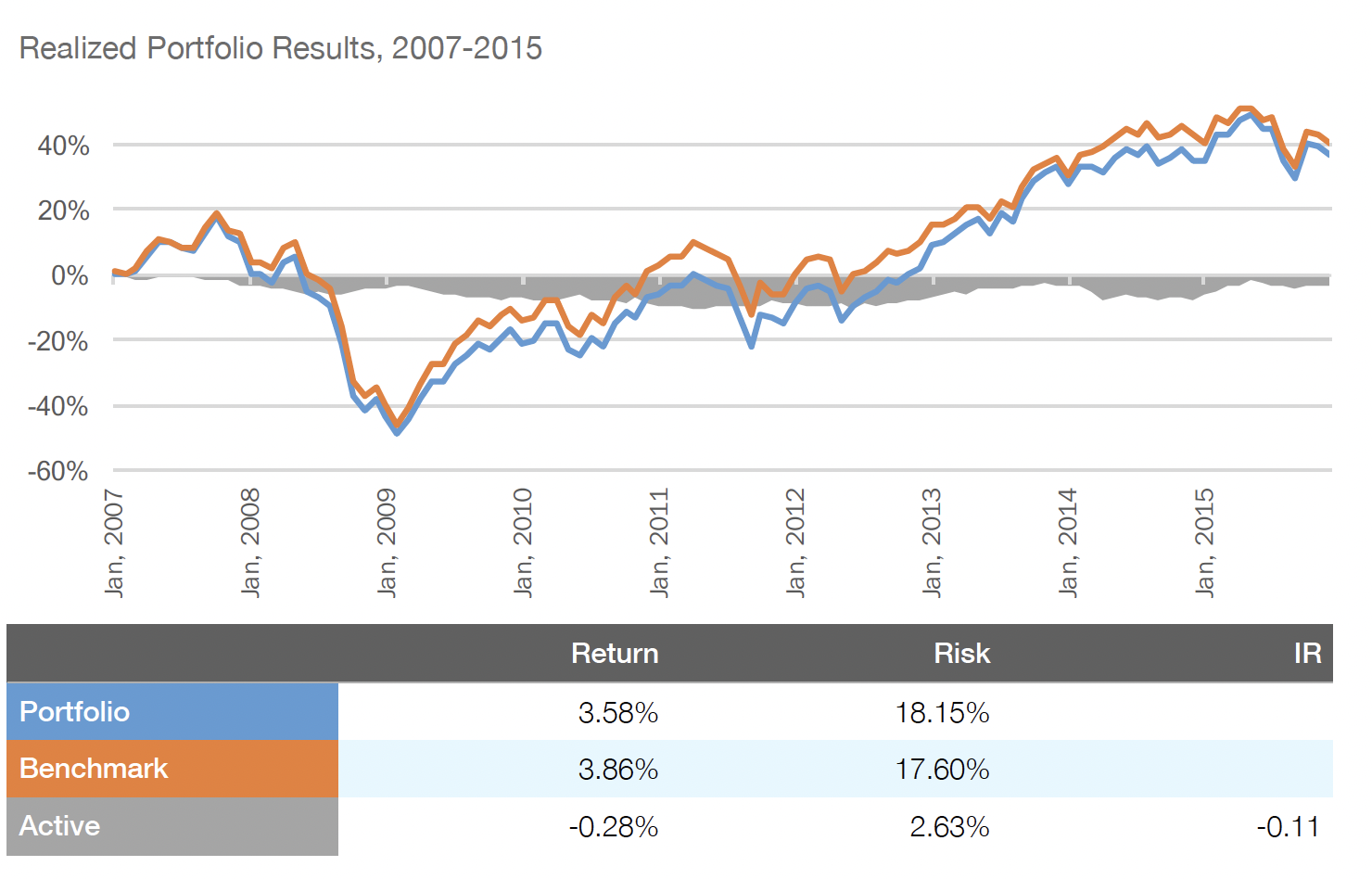
As you can see, the fund underperformed its benchmark, the FTSE All- World Index, and at the same time was more risky. Though, when we use Axioma’s WW4 factor risk model to breakout the stock-specific performance versus the factor performance, we see an interesting story emerge.
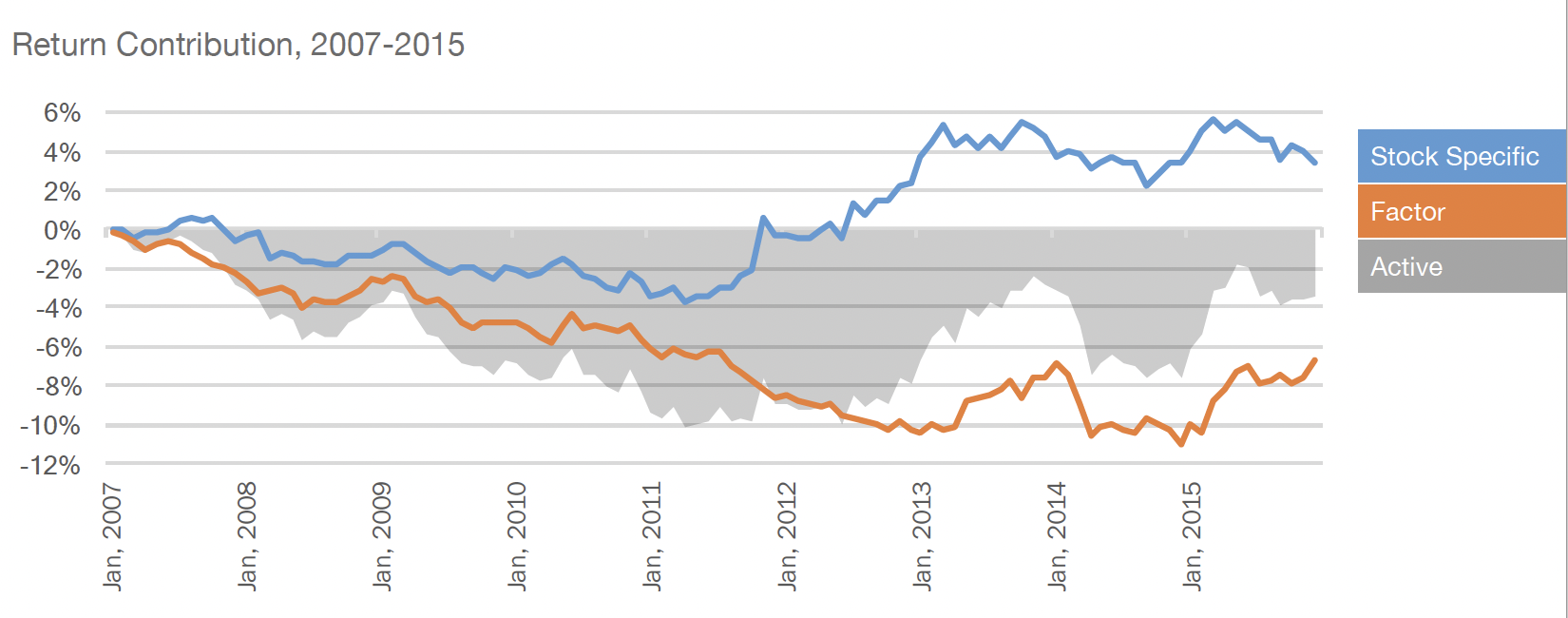
This PM has a winning alpha strategy, but factor contribution has a large negative effect. We then breakdown the factor and alpha piece even further:
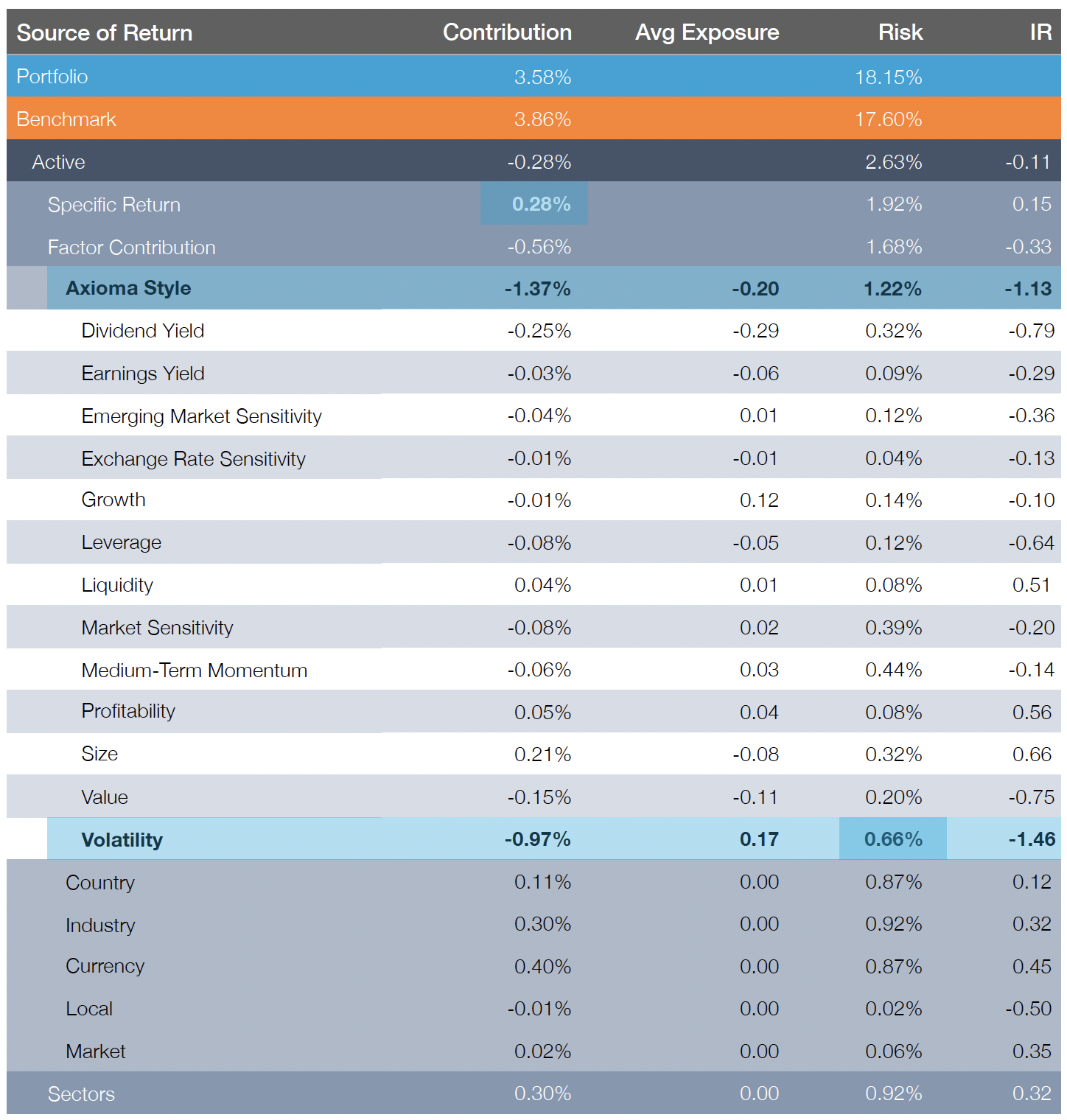
When looking for sources of underperformance, we first see that specific return is positive, at +0.28%. This indicates that the stock-specific selection alpha is working as intended. Without alpha from stock selection, our quantitative adjustment would not make sense. Next, we see that the bulk of the underperformance comes from the ‘Axioma Style’ factors, and ‘Volatility’ in particular. This bottom-up fund was not just making a bet on the stocks picked by their analysts and portfolio managers, they were also making bets on global risk factors, which were intrinsic to their stock selection and weighting decisions.
We use our factor model to break down the fund’s ex ante risk over time:
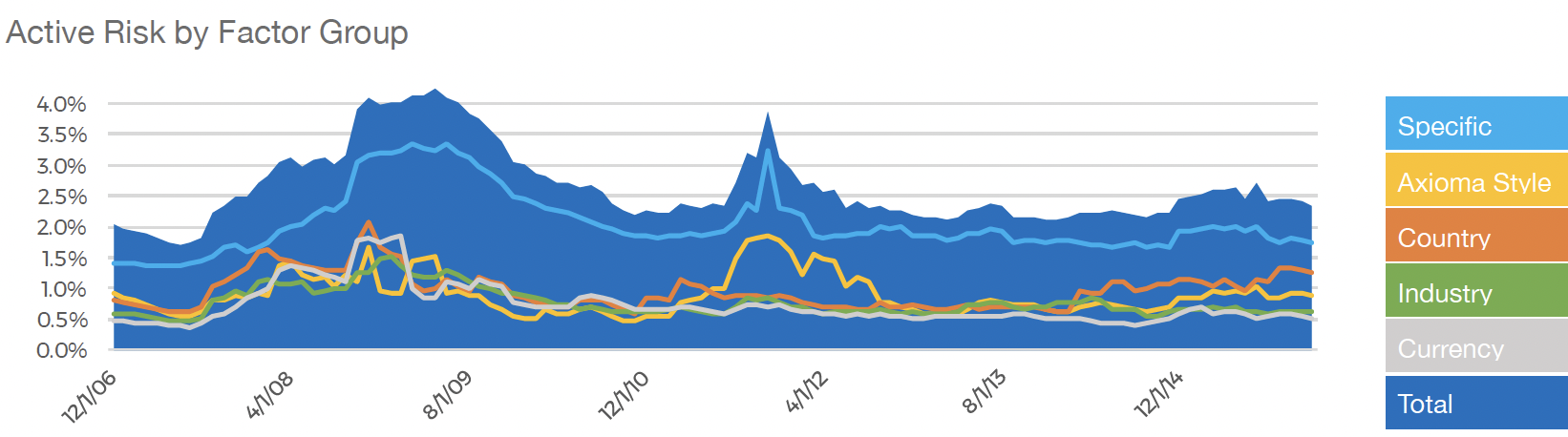
The largest active risk contribution is from specific risk, and this is exactly the type of risk a fundamental bottom-up stock picker wants. Their risk policies are working well. However, there is still room for improvement as there are periods when Axioma Style, Country, Industry and Currency exposures still add significant risk to the portfolio. We see that factor exposures often account for over half of this fund’s active risk.
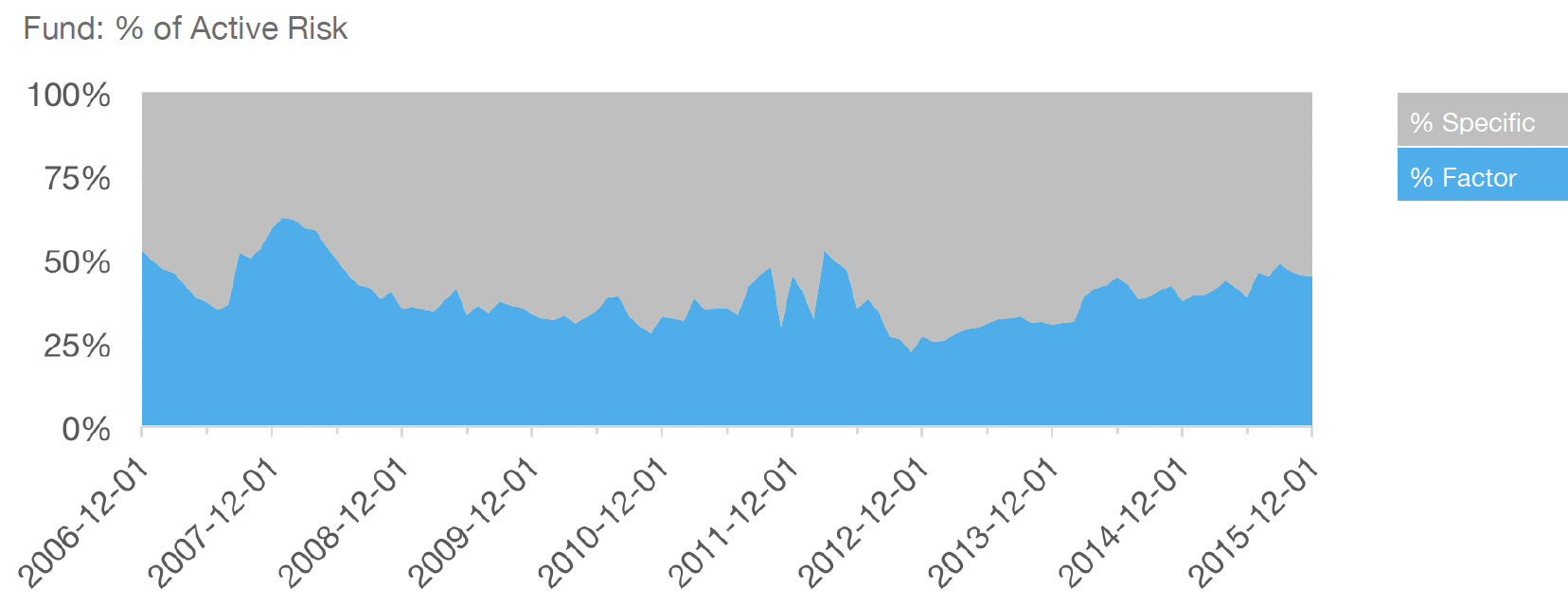
It’s feasible but difficult to reduce the return of factor contribution manually. It would involve a lot of trial and error; stocks with undesirable factor exposures would have their portfolio weighting tweaked, and after each potential change an analysis of ex ante risk can be run. It’s far easier to run an optimizer that will take into account not only the factor exposures and correlations, but also critical portfolio constraints in order to come up with a portfolio that meets the defined criteria.
One important optimization criteria is minimizing the change in portfolio weightings. An equity’s portfolio weighting is chosen with specific risk- reward scenarios in mind. Adding a new stock solely due to desirable factor risk exposures, scaling up a small position or minimizing the size of their highest conviction position are not actions any sane portfolio manager would take. We set out four alternative rebalancing criteria, including the “No Limit” scenario, as an illustrative example as to why optimizers are given reasonable constraints.
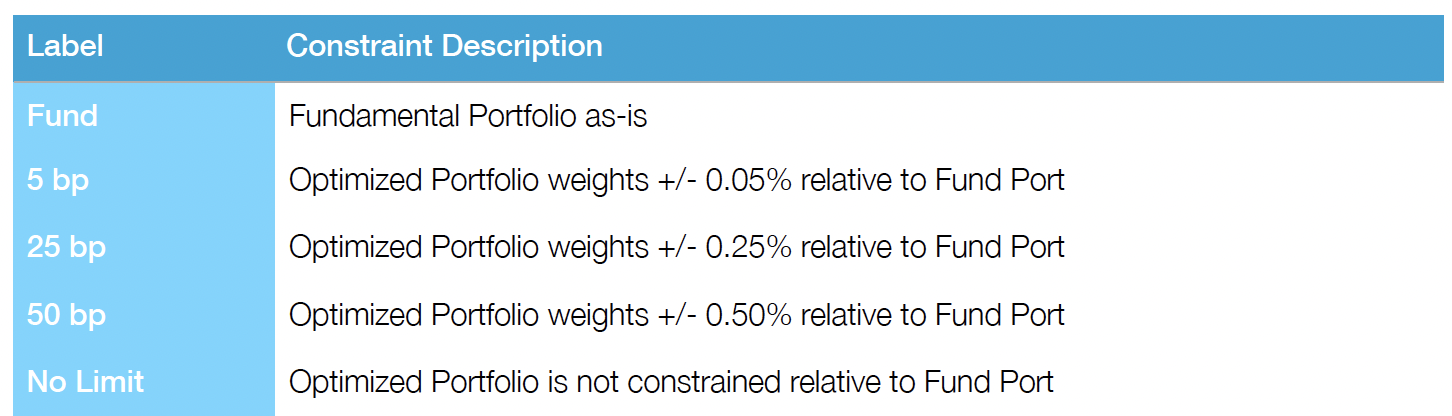
We did allow the portfolio to set some stock holding weightings to zero if the stock’s weight was small enough to begin with. The names held varied over time, with the most volatility found in the unconstrained optimization.
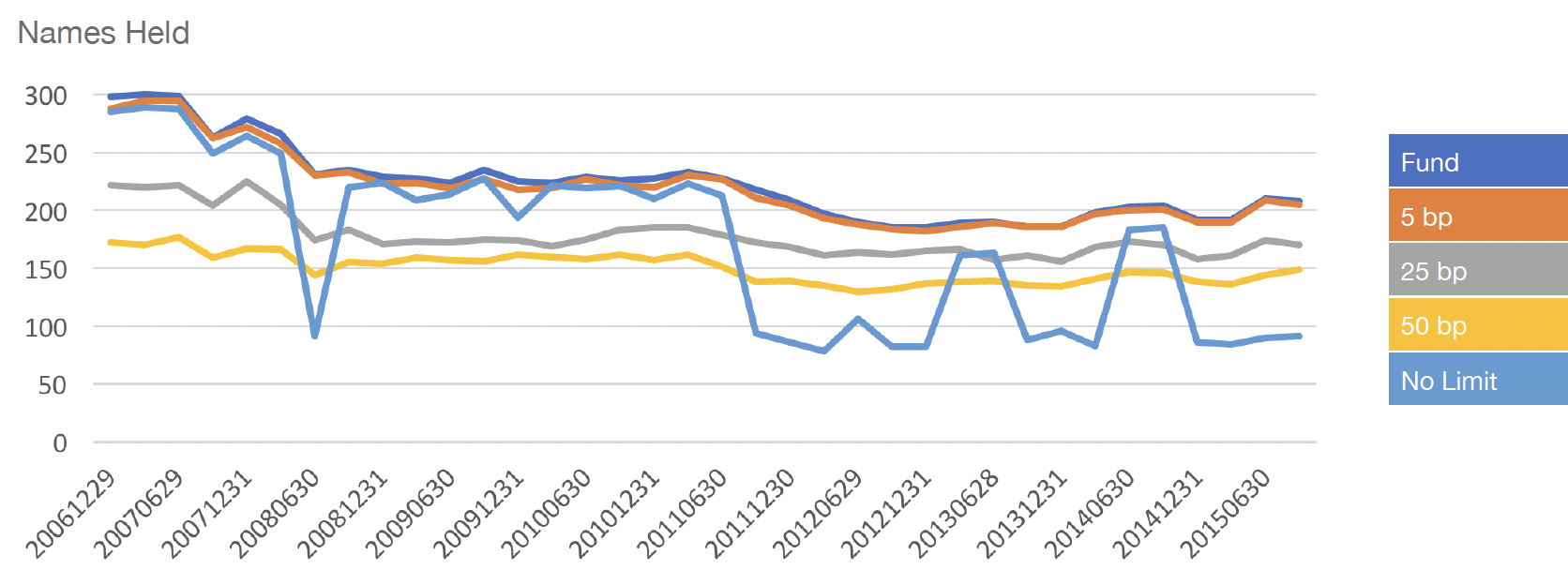
Allowing unconstrained limits changes the portfolio significantly, with the other optimization criteria generating historical portfolios that are far more similar to the original stock holdings. Not surprisingly, the tighter the constraints, the greater the correlation between portfolio holdings.
When we look at the turnover required to create each portfolio, without using the optimizer’s turnover constraints, the turnover of the Fund acts as a floor.
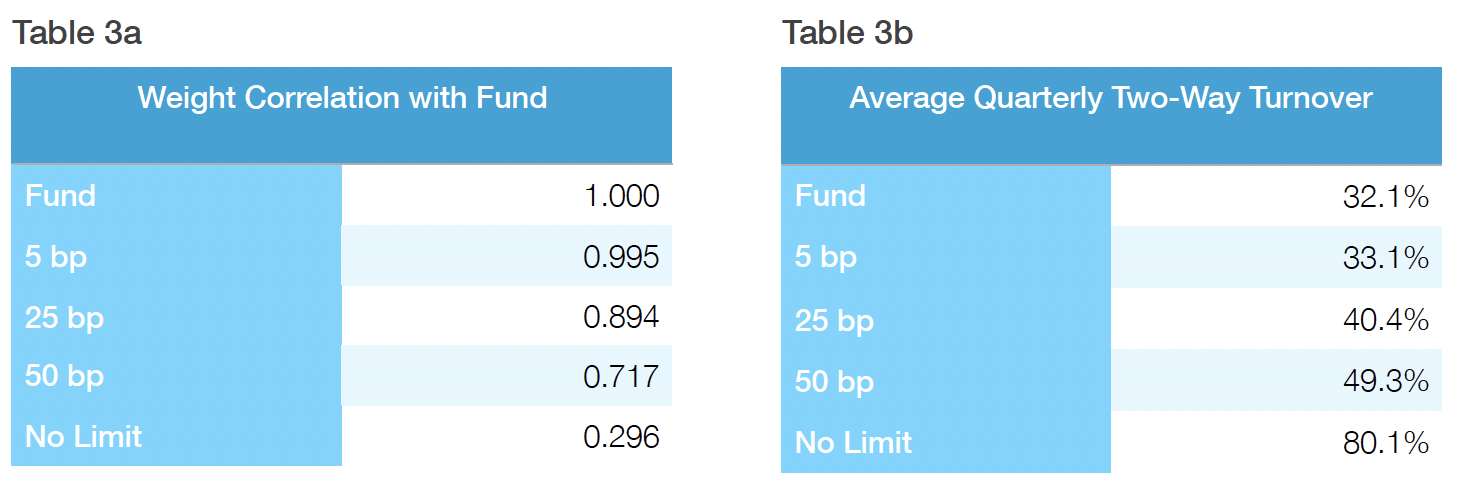
Every investor knows that too many transactions can sink a portfolio’s performance, so we added a new portfolio constraint, a 25bp weighting constraint combined with a turnover constraint to match that of the fund.
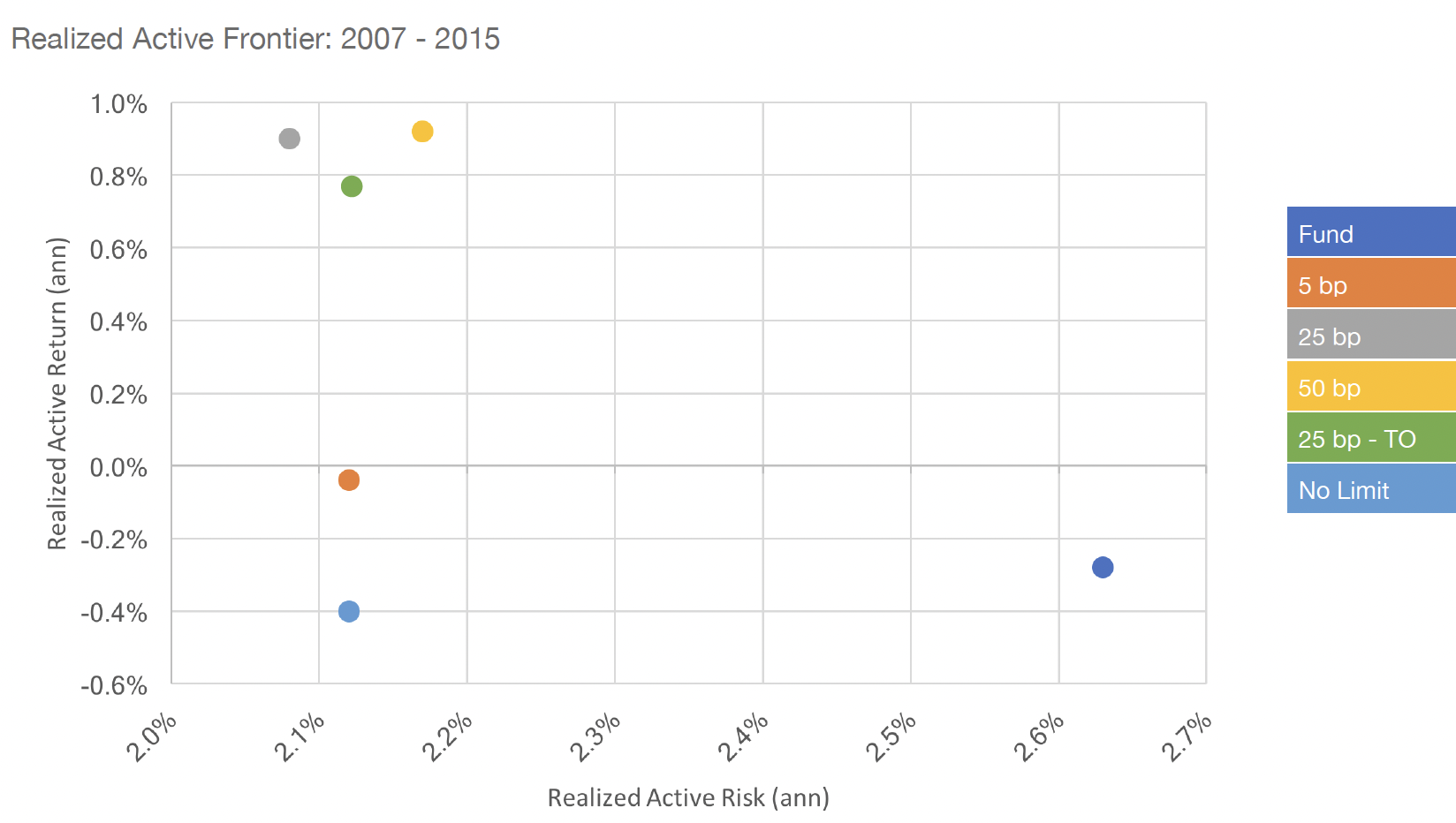
When we look at the results, the 5 bp, 25 bp and 50 bp portfolios all outperformed the original portfolio. Placing no limit on the portfolio weighting changes caused active returns to be lower than pre- optimization. Adding the turnover constraint to the 25 bp optimization reduced the active returns, but should increase performance overall as these numbers are pre-transaction costs.
When we take a deeper dive into the optimized portfolio that keeps the fund’s stock holdings within their original 25 bp target and retains the exact same turnover as the original Fund Port, we see materially different risk & return decomposition from the original.
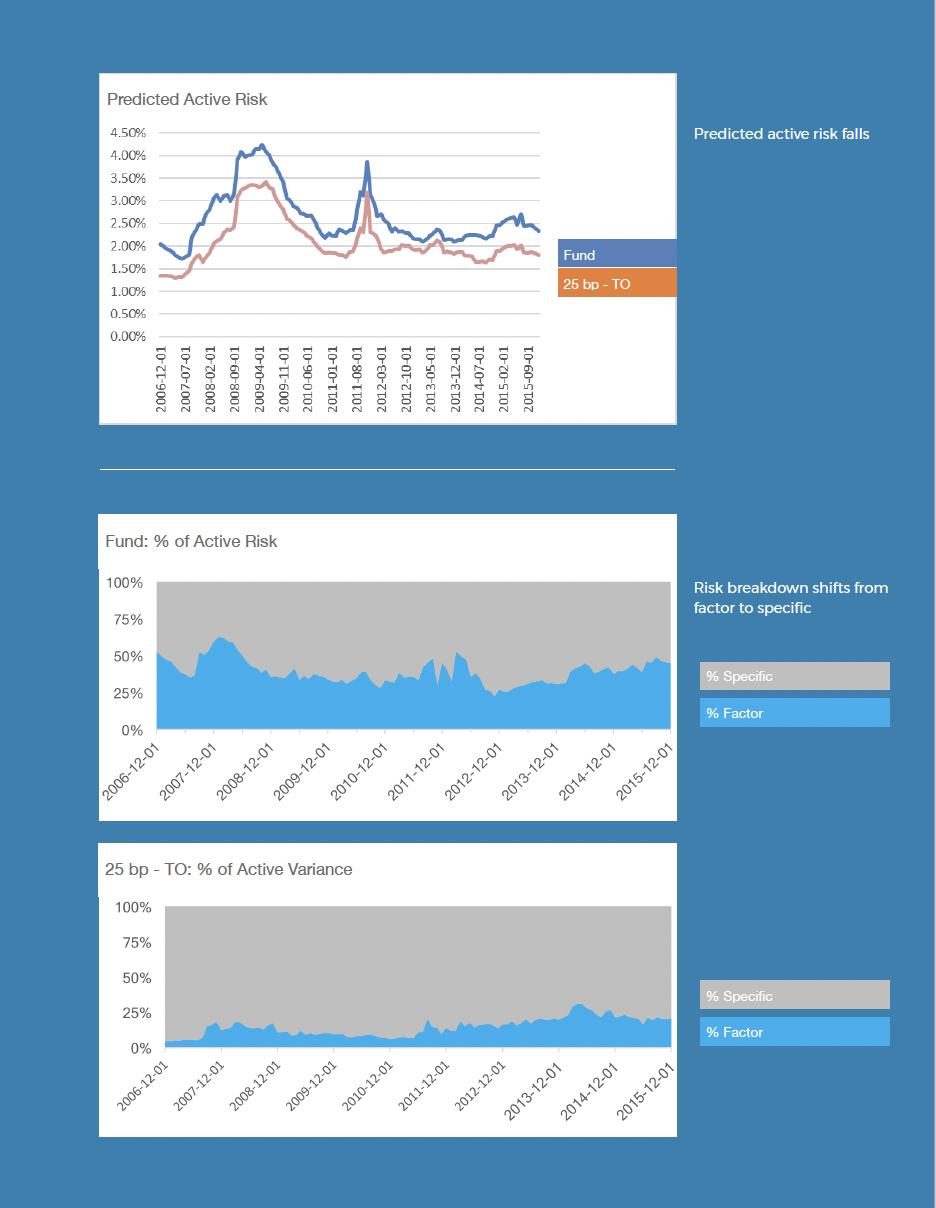
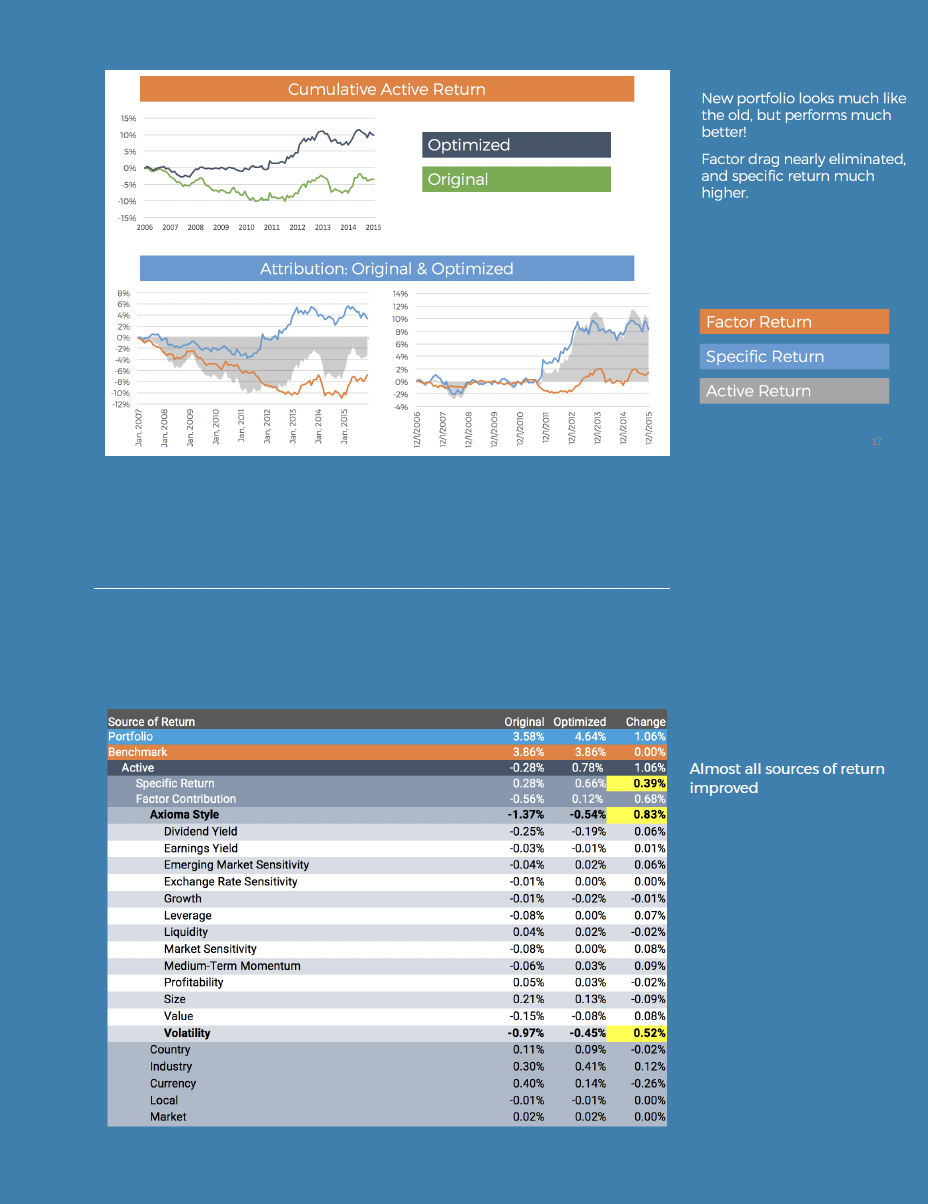
The optimization ultimately reduced the style risk. The largest performance improvement came from reducing the risk allocated to the volatility factor, reducing its drag on the fund from 0.97% to 0.45%. Overall, the changes allowed for the creation of a portfolio that beat its benchmark rather than marginally underperforming.
The optimization done on this portfolio was a very basic one. We were only trying to minimize factor-specific risk relative to the FTSE All-World index using very simple heuristics. In practice, a portfolio manager would use other aspects of the optimizer, such as giving stocks conviction scores to ensure that high conviction stocks do not get down weighted in favor of low conviction stocks. Even absent that, its notable that a little bit of factor optimization could have helped this fundamental manager turn underperformance into outperformance by maximizing the impact of their ability to pick stocks.
Conclusion
Every portfolio manager understands the need for portfolio constraints. And not every manager has the same portfolio constraint. Different metrics are applied to managers tracking different benchmarks. The Factor Contribution is another way of quantifying risk constraint. Some exposures are desirable, others should be avoided. No portfolio manager would let their technology exposure drift outside of their target range without knowing they were making a very specific choice. This fund let active risk from the volatility factor alone reach 0.66%. That ended up costing 0.97% in active return.
Technical Appendix: Quantitative Tools
Factor Risk Models
Factor risk models are a powerful tool that helps investment professionals on three fronts: ex- ante risk, ex-post attribution of returns and risks, and portfolio construction.
Factor risk models are an ex-ante tool to help investment professionals understand the sources of predicted risk and realized risk and return in a portfolio. From a predicted risk perspective, factor risk models provide a predicted standard deviation of active returns given a portfolio and a benchmark, and decomposes the sources of those risks across both systematic (i.e., factor) components and an idiosyncratic (i.e. specific) component. As a concrete example, Axioma’s WW4 model includes several different factor blocks - Style factors, Industry factors, Country factors, Currency factors, and a Market factor – along with a specific risk model.
The various factor blocks help quantify commonalities across assets in the market, so we can better understand what risks assets are exposed to. Style factors include both factors comprised of market based measures such as Medium-Term Momentum, Size, and Volatility, as well as balance sheet based measures such as Value, Leverage, and Growth. Assets with a high Value score behave differently than assets with a low Value score, and the risk model accordingly captures this behavior. Industry factors are driven by the GICS industry mapping of the asset, Country factors by the country membership of the asset, and Currency factors by the currency denomination of the asset. Assets with a common Industry will generally behave much more similarly than assets with a dramatically different Industry, and again the risk model accordingly captures this behavior.
Every asset has an exposure to every one of these various factors and each factor has its own behavior and correlation with other factors. Any behavior that is not captured by these various factors is considered ‘idiosyncratic risk’ or ‘specific risk’ – the risks that stem from the unique business model of the company itself which is not common across the broad market.
From an ex-post perspective, Factor risk models allow finance professionals to understand what drove their portfolio’s realized returns and risks and help confirm their performance is in line with their investment process. These realized returns and risks can be performed using a Factor Risk model, which helps decompose realized results across the various factor blocks and the specific block. Just as in the view of risk through the lens of the risk model, having the reports be consistent with one’s investment process is critical. The factor-based performance attribution paper can also help one discover if factors are helping or hurting realized performance, so managers can make better informed decisions with respect to managing their portfolio on an ongoing basis.
As a fundamental manager picking stocks, stock Specific risk and returns are preferred to Factor risks and returns. Stock Specific risk and return is also known as ‘alpha’ and delivers value that factor-based Smart Beta products and Quants don’t always deliver.
Portfolios with large amounts of Specific risk are what most limited partners expect from the investment approach of a bottom-up Fundamental manager, so that is what we maximized with our optimizer in this case study.
Optimizers
Optimizers can help bottom-up Fundamental managers make slight changes to their asset weights to tilt their portfolio to desired risks and away from undesired risks.
At a high level, optimizers are simply a tool to help make better decisions – which can apply to almost any facet of life, not just finance. At the core of any optimization is a goal one is trying to achieve, while obeying certain rules we cannot violate. Optimizers are best known in the finance world from Markowitz’s Mean Variance optimization framework, where the goal is to maximized expected returns less variance. In this case, the ‘variance’ is quantified by a risk model, which can be a factor risk model or a simply covariance matrix quantifying asset-asset interactions.
But Axioma’s optimizer does not force you into the Mean-Variance optimization space, which wouldn’t make sense for a fundamental Manager who is not building quantitative expected returns. In the case of a Fundamental manager, the ‘buy list’ of assets with varying conviction is known along with an idea of an initial portfolio weighting based on the level of conviction. But this initial portfolio based on the level of conviction may be heuristic based and not necessarily be ‘tuned’ to load up on Specific risk or other desired Factor risks and minimize undesired Factor risks. We are therefore faced with a challenge of staying relatively close to the initial portfolio so we maintain high weights in high conviction assets, while attempting to remove our unwanted factor risks.
There are two main algorithms to Axioma’s optimizer: a ‘Second Cone Order Program’ (SOCP) and a ‘Mixed Integer Program’ (MIP).
SOCP is the next evolution of optimizers beyond a Quadratic Program (popularized by Markowitz used in Mean-Variance optimization). There are no iterations with Axioma. Axioma’s optimizer defines the optimization problem mathematically and is able to find the mathematically provable best solution. SOCP handles the differentiable parts of the optimization – i.e. the ‘smooth’ part of the optimization.
MIP is a modeling layer on top of the SOCP, which allows for the solving of real world discrete constraints such as: Limit Number of Names, Limit Number of Trades, Threshold Holding/Trade (i.e. hold/trade x basis points, or don’t trade it at all). These constraints are very hard to solve for, and it’s Axioma’s specialty. Given the combinatorial nature of these problems, we essentially build decision trees to ‘search’ the various combinations of solutions and trim down the bad options and focus our search on the good ones (good = better objective value). In this case, we don’t always end up with the optimal solution (as some optimal solutions could take a very long time to find) but we quantify how far off a given portfolio is from the optimal solution.
For this specific case study, we only used the SOCP.
Appendix 2: Axioma Style Factors Explained
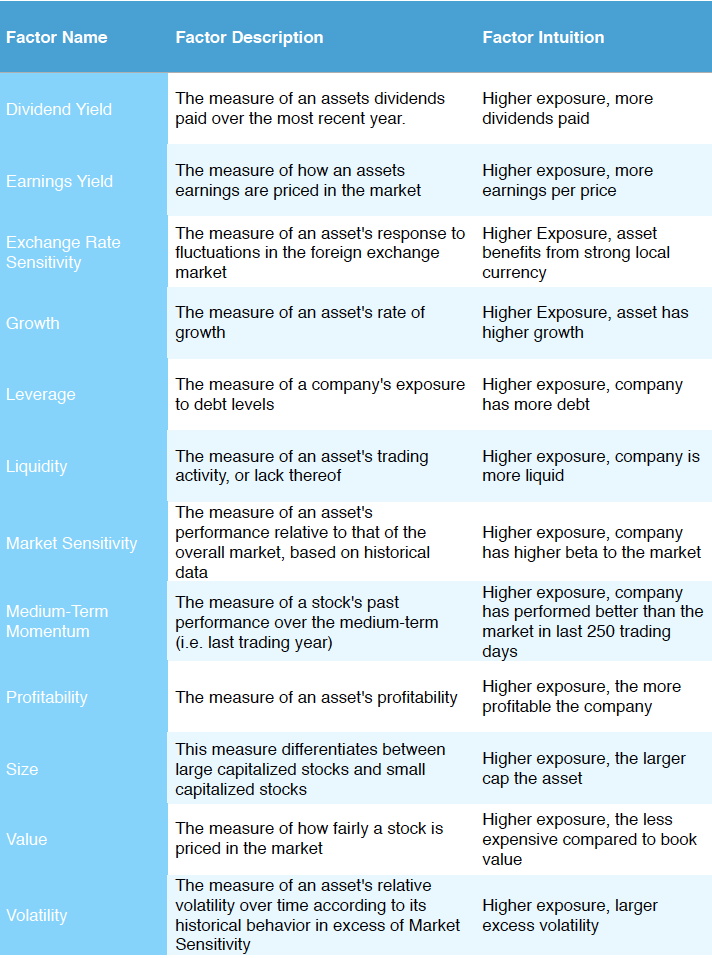